Keynote Speakers
Weisi Lin
Title: New Opportunities of Visual Representation for Machine Intelligence
Abstract: With the waves of AI, increasingly more visual signals are intended for machines (rather than humans) as the ultimate users. What opportunities may this shift bring for visual representation, e.g., to address the requirements of Video Coding for Machines (VCM), JPEG AI and beyond? In this talk, we will first discuss how to determine visual signal sensitivity toward machine intelligence (MI). MI-oriented models can be also developed for identity/privacy protection. Secondly, a possible paradigm change of visual representation is explored: intermediate, deep-learnt visual features (instead of a whole image) can be the basic unit of representation for MI. This brings intelligence to the edge, facilitates edge-cloud collaboration, and leads to integration of signal representation and computer vision which have been separate processes for long.
Short Bio: Weisi Lin researches in intelligent image and video processing, computational perceptual signal assessment, and multi-modality/media modeling. He received his B.Sc and M. Sc from Sun Yat-Sen University, China, and Ph.D. from King’s College, U.K. He is currently a Professor in School of Computer Science and Engineering, Nanyang Technological University, Singapore, where he also serves as the Associate Chair (Research).
He is a Fellow of IEEE and IET, and has been a Highly Cited Researcher 2019, 2020 and 2021. He has elected as a Distinguished Lecturer in both IEEE Circuits and Systems Society (2016-17) and Asia-Pacific Signal and Information Processing Association (2012-13), and given keynote/invited/tutorial/panel talks in 40+ international conferences. He has been an Associate Editor for IEEE Trans. Image Process., IEEE Trans. Circuits Syst. Video Technol., IEEE Trans. Multimedia, IEEE Signal Process. Lett., Quality and User Experience, and J. Visual Commun. Image Represent., and a Senior Editor in APSIPA Trans. Info. and Signal Process, as well as a Guest Editor for 7 special issues in different journals. He also chaired the IEEE MMTC QoE Interest Group (2012-2014); he has been a Technical Program Chair for IEEE ICME 2013, QoMEX 2014, PV 2015, PCM 2012 and IEEE VCIP 2017. He leads the Temasek Foundation Programme for AI Research, Education & Innovation in Asia, 2020-2025. He believes that good theory is practical, and has delivered 10+ major systems for industrial deployment with the technology developed.
Personal website: https://personal.ntu.edu.sg/wslin/Home.html
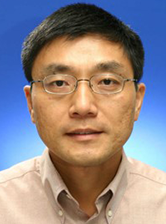
Prof. Kyoung Mu Lee
Director of Interdisciplinary Program in Artificial Intelligence
Dept. of ECE, Seoul National University
Title: Task Adaptive Meta-Learning for Computer Vision
Abstract: Few-shot learning is an emerging yet challenging problem in which the goal is to achieve generalization from only a few examples. Meta-learning tackles few-shot learning via learning prior knowledge shared across tasks and using it to learn new tasks. Owing to the flexibility and generalizability, optimization-based meta-learning algorithms have emerged as one of the promising methods for few-shot learning. MAML is one of the most popular instances of optimization approaches, owing to its simplicity and applicability across diverse problem domains. However, it is known for its low generalization performance. To overcome the limitations of the MAML-based methods, we introduce effective and flexible optimization-based meta-learning algorithms that are generalizable across diverse practical domains. More specifically, to achieve outstanding and robust performance, novel task-adaptive optimization schemes (task-adaptive initialization, task-adaptive update-rule, and task-adaptive loss function) are introduced to more effectively facilitate the adaptation to diverse tasks. The extensive experiments have demonstrated the outstanding performance of proposed meta-learning algorithms with task-adaptive optimization across few-shot learning benchmark datasets and more realistic computer vision problems such as visual tracking and video frame interpolation.
Short Bio: KYOUNG MU LEE (Fellow, IEEE) is currently the Editor in Chief of the IEEE TRANSACTIONS ON PATTERN ANALYSIS AND MACHINE INTELLIGENCE (TPAMI); He received the B.S. and M.S. degrees in control and instrumentation engineering from Seoul National University (SNU), Seoul, South Korea, in 1984 and 1986, respectively, and the Ph.D. degree in electrical engineering from the University of Southern California, in 1993. He is the director of the Interdisciplinary Graduate Program in Artificial Intelligence at SNU. He is an Advisory Board Member of the Computer Vision Foundation (CVF). He was a Distinguished Lecturer of the Asia-Pacific Signal and Information Processing Association (APSIPA), from 2012 to 2013. He has received several awards, in particular, the Medal of Merit and the Scientist of Engineers of the Month Award from the Korean Government, in 2018 and 2020, respectively; the Most Influential Paper Over the Decade Award by the IAPR Machine Vision Application, in 2009; the ACCV Honorable Mention Award, in 2007; the Okawa Foundation Research Grant Award, in 2006; the Distinguished Professor Award from the College of Engineering of SNU, in 2009; and the SNU Excellence in Research Award in 2020. He has also served as a General Chair for ICCV2019, ACMMM2018, and ACCV2018; a Program Chair for ACCV2012; a Track Chair for ICPR2020 and ICPR2012; and an Area Chair for CVPR, ICCV, and ECCV many times. He has served as an Associate Editor-in-Chief (AEIC) and an Associate Editor for the Machine Vision and Application (MVA) journal, the IPSJ Transactions on Computer Vision and Applications (CVA), and the IEEE SIGNAL PROCESSING LETTERS (SPL); and an Area Editor for the Computer Vision and Image Understanding (CVIU). He is the founding member and served as the President of the Korean Computer Vision Society (KCVS). Prof. Lee is a Fellow of IEEE, a member of the Korean Academy of Science and Technology (KAST) and the National Academy of Engineering of Korea (NAEK).
Personal website: https://cv.snu.ac.kr/index.php/~kmlee/
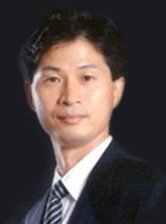
Dr. Dong Yu
Title: Voice Enhancement, Separation, and Compression
Abstract: We have seen significant progress in voice processing in the past several years. In this talk, I will introduce a series of techniques developed at Tencent AI Lab on voice enhancement, separation, and compression and their applications in real-world scenarios such as music separation, in-car voice processing, and online meetings. I will describe the key paradigm change behind all these advancements and the solutions to new problems observed in the new paradigm.
Short Bio: Dr. Dong Yu is an IEEE Fellow, an ISCA Fellow, and an ACM distinguished scientist. He currently works at Tencent AI Lab as a distinguished scientist and vice general manager. Prior to joining Tencent in 2017, he worked as a principal researcher at Microsoft Research (Redmond), where he had been since 1998. He has concentrated his research on speech recognition and processing, as well as natural language processing in recent years. He has two monographs and over 300 papers to his credit. His work has been widely cited, and he has received the prestigious IEEE Signal Processing Society best paper award in 2013, 2016, and 2020, as well as the 2021 NAACL best long paper award. He was a forerunner in the use of deep learning techniques in automatic speech recognition.
Dr. Dong Yu is currently serving as the chair of the IEEE Speech and Language Processing Technical Committee (SLTC). He has served on the editorial boards of numerous journals and magazines, as well as on the organizing and technical committees of numerous conferences and workshops, including serving as the technical co-chair of ICASSP 2021.
Personal website: https://sites.google.com/view/dongyu888/
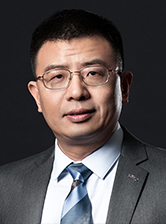