APSIPA ASC 2020: Overview Session
Day-1 (December 8) |
Session 1-1 12:30~14:00 |
Chair: Prof. Woon-Seng Gan
|
Talk 1 :
Speaker: Prof. Christian Ritz
Affiliation: University of Wollongong (UOW), Australia
Talk Title: Co-prime Microphone Arrays and their Application to Finding the Direction of Arrival of Sound Sources
Talk 2 :
Speaker: Prof. Hiroshi Saruwatari
Affiliation: University of Tokyo, Japan
Talk Title: Multichannel audio source separation based on unsupervised and semi-supervised learning
Talk 3 :
Speaker: Prof. Woon-Seng Gan
Affiliation: Nanyang Technological University, Singapore
Talk Title: : Signal Processing an AI for Spatial Augmented Reality Audio (SARA): Sensing, Control and Rendering
|
Session 1-2 15:30~17:00 |
Chair: Prof. Hitoshi Kiya
|
Talk 1 :
Speaker: Prof. Hitoshi Kiya
Affiliation: Tokyo Metropolitan University, Japan
Talk Title: Image transformation for privacy-preserving machine learning and adversarially robust defense
Talk 2 :
Speaker: Prof. Nam Ik Cho
Affiliation: Seoul National University, Korea
Talk Title: Recent Issues in Real-World Image Restoration Using Deep Convolutional Neural Networks
Talk 3 :
Speaker: Prof. Chris Gwo Giun Lee
Affiliation: National Cheng Kung University (NCKU), Taiwanv
Talk Title: : Machine Learning for Analytics Architecture: AI to Design AI
|
Day-2 (December 9) |
Session 2-1 12:30~14:00 |
Chair: Prof. Chi-Chun Lee (Jeremy)
|
Talk 1 :
Speaker: Prof. Hemant A. Patil
Affiliation: DA-IICT Gandhinagar, India
Talk Title: Spoofing Attacks in Automatic Speaker Verification (ASV): Analysis and Countermeasures (This talk is also an ISCA Distinguished Lecture)
Talk 2 :
Speaker: Prof. Qin Jin
Affiliation: Renmin University, China
Talk Title: : Natural Language Description of Image and Video Content
Talk 3 :
Speaker: Prof. Chi-Chun Lee (Jeremy)
Affiliation: National Tsing Hua University (NTHU), Taiwan
Talk Title: Enabling in-to-life Emotion-AI technology: robustness, scalability, and trustworthiness
|
Session 2-2 15:30~16:30 |
Chair: Prof. Xudong Jiang
|
Talk 1 :
Speaker: Prof. Xudong Jiang
Affiliation: Nanyang Technological University (NTU), Singapore
Talk Title: Semantic Image Scene Segmentation by Deep Machine Learning
Talk 2 :
Speaker:Prof. Yuchao Dai
Affiliation: Northwestern Polytechnical University, Xi'an, China
Talk Title: Towards Comprehensive Understanding of 3D: Geometric Computer Vision Meets Deep Learning
|
IMAGE AND VIDEO OVERVIEW SESSION
Image transformation for privacy-preserving machine learning and adversarially robust defense
Abstract:
Although the rapid development of machine learning algorithms has led to major breakthroughs in computer vision, for a wide range of applications, where safety and security are critical, there is concern about the reliability of machine learning systems. Such systems in general suffer from untrusted environments and various attacks such as adversarial attacks, unauthorized use of data, model inversion attacks, and membership inference attacks.
Accordingly, various image transformation methods have been proposed for privacy-preserving machine learning and adversarially robust defense. In contrast, attack methods on such image transformation methods have been investigated simultaneously. In my talk, we first address compressible image encryption schemes, which have been proposed for encryption-then-compression (EtC) systems, and their application to traditional machine leaning algorithms such as support vector machine. Next, image transformation methods including perceptual encryption ones that generate images without visual information are summarized for privacy-preserving DNNs. Finally, block-wise image transformation methods with a secret key is demonstrated to be able to maintain a high classification accuracy under the use of both clean images and adversarial examples.
Biography:
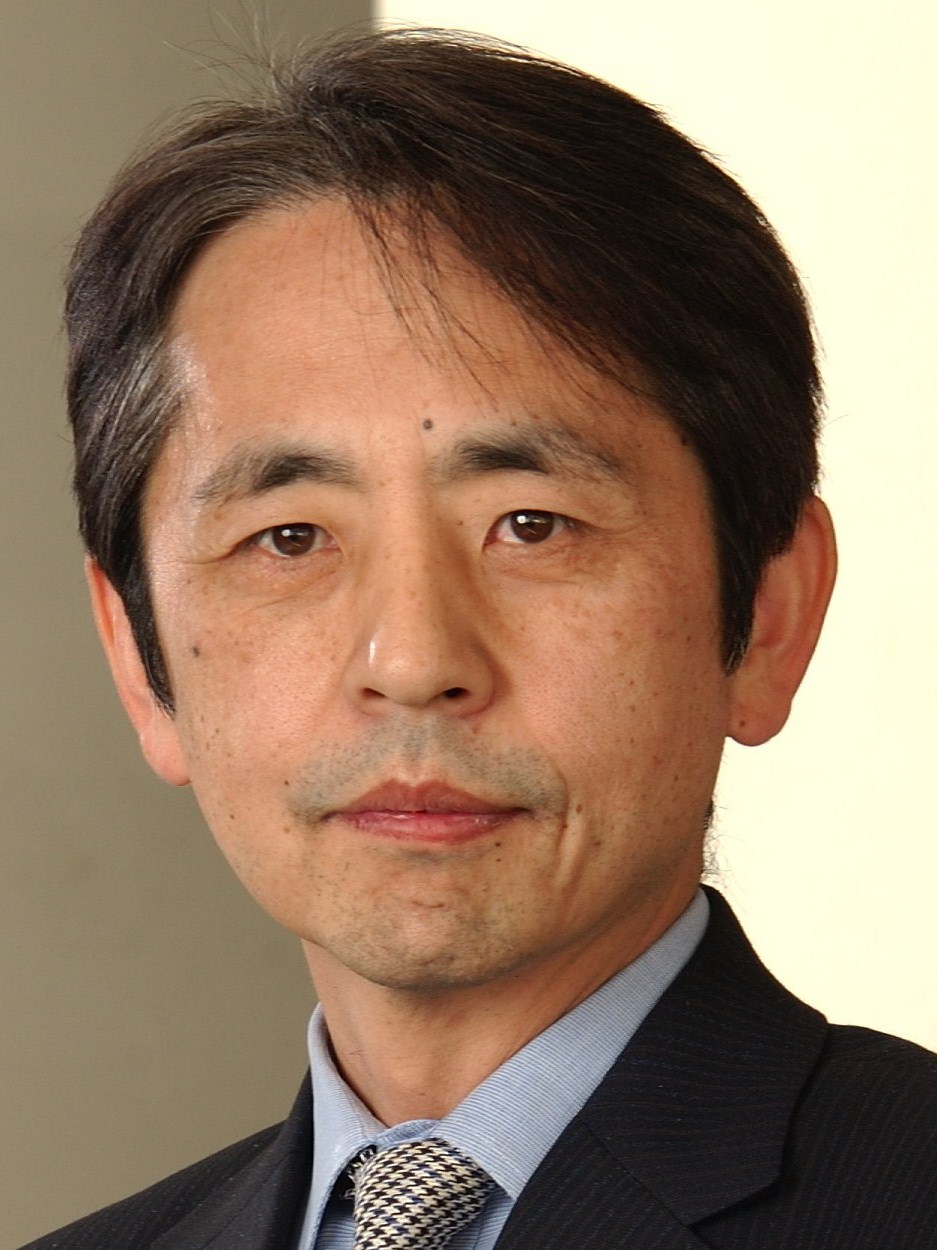
HITOSHI KIYA received the B.E. and M.E. degrees from the Nagaoka University of Technology, Japan in 1980 and 1982, respectively, and the Dr.Eng. degree from Tokyo Metropolitan University, in 1987. In 1982, he joined Tokyo Metropolitan University, where he became a Full Professor, in 2000. From
1995 to 1996, he was a Visiting Fellow with The University of Sydney, Australia. He served as the Inaugural Vice President (Technical Activities) of APSIPA, from 2009 to 2013, and the Regional Director-at-Large for Region ten of the IEEE Signal Processing Society, from 2016 to 2017. He was also the President of the IEICE Engineering Sciences Society, from 2011 to 2012. He currently serves as the President of APSIPA. He is a Fellow of IEEE, IEICE and ITE. He has received numerous awards, including ten best paper awards. He has organized a lot of international conferences in such roles as the TPC Chair of the IEEE ICASSP 2012 and the General Co-Chair of the IEEE ISCAS 2019. He was an Editorial Board Member of eight journals, including the
IEEE Transactions on Signal Processing, Image Processing, and Information Forensics and Security.